The Ultimate Guide to Video Labelling Tools for Enhanced Data Annotation
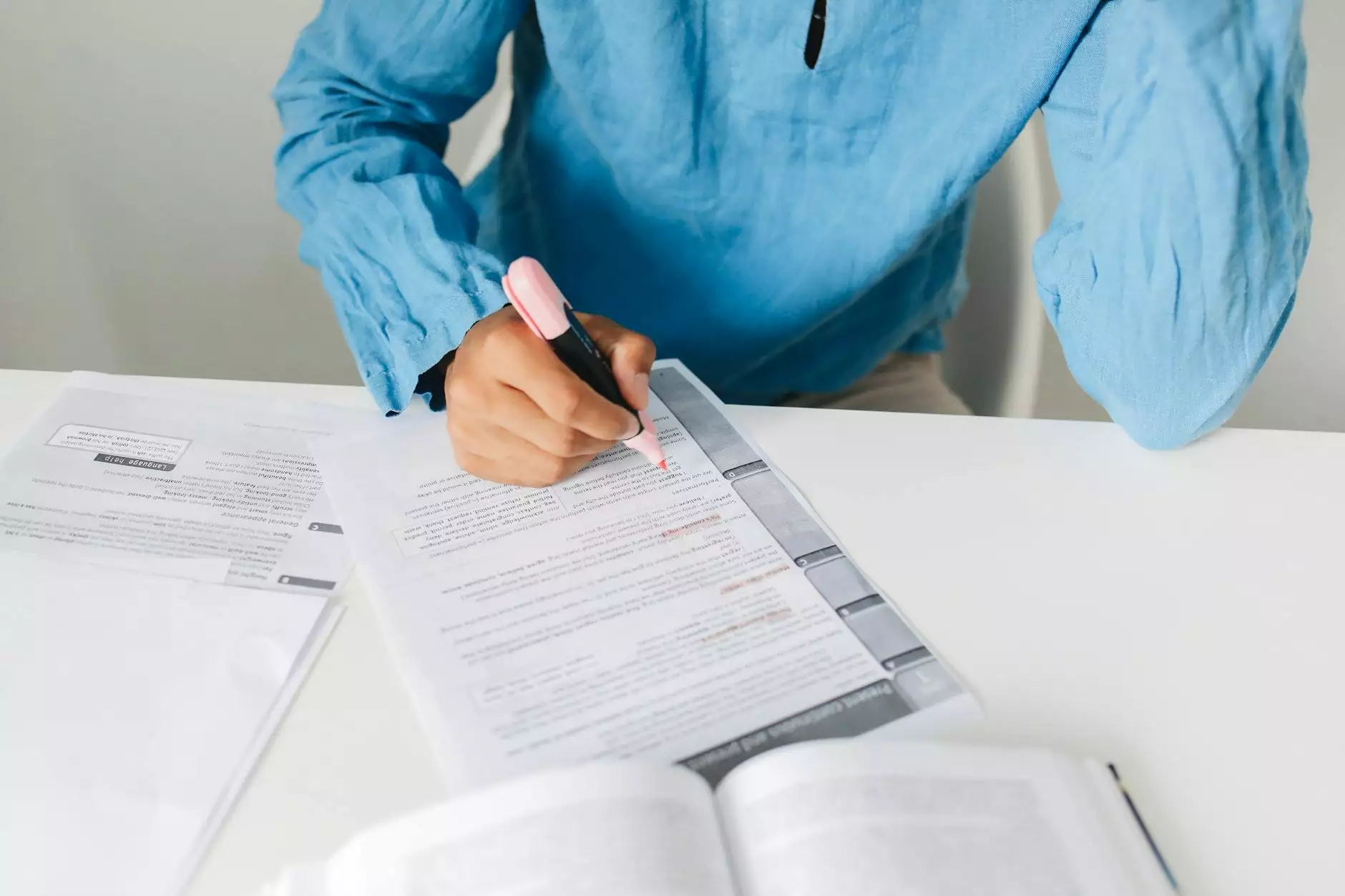
In today’s fast-paced digital world, the process of data annotation is rapidly becoming one of the most crucial elements in the fields of artificial intelligence and machine learning. Among the various types of data, video data is particularly significant due to the rise of applications that utilize video content, from autonomous vehicles to surveillance systems. To effectively leverage this data, businesses are investing heavily in video labelling tools, which facilitate the organization and analysis of video data.
Understanding Video Labelling Tools
Video labelling tools are software applications designed to aid in the process of annotating video content. These tools allow users to add metadata to specific parts of a video, enabling machine learning models to learn from the labelled data. By using video labelling software, organizations can streamline their data processing workflows, enhance the accuracy of their models, and ultimately make more informed decisions based on high-quality annotated data.
The Importance of Data Annotation
Data annotation is critical for training AI models and improving their performance. When it comes to video data, the intricacies involved make it even more essential. Without properly labelled data, algorithms may struggle to recognize objects, actions, and events occurring within video footage. Consider the following benefits of effective video annotation:
- Accuracy in Model Predictions: Properly annotated data leads to better training input for machine learning models, resulting in improved accuracy and reliability.
- Efficiency in Workflows: Automated video labelling tools can significantly reduce the time taken for data processing, allowing teams to focus on higher-value tasks.
- Enhanced Decision-Making: With well-annotated data, organizations can gain insights that drive strategic decisions and operational improvements.
Key Features of Effective Video Labelling Tools
When selecting a video labelling tool, it's essential to opt for one that possesses a robust set of features to maximize your data annotation efforts. Here are some key features to consider:
1. User-Friendly Interface
A straightforward and intuitive interface allows users to navigate and annotate video content efficiently. This is crucial for training team members, as a complex interface can hinder productivity.
2. Support for Multiple Formats
Look for a tool that supports various video formats to ensure compatibility with different sources. This flexibility helps in integrating videos from various platforms.
3. Automation Capabilities
Advanced video labelling tools offer automation features, such as auto-tagging and smart suggestions. These capabilities can drastically reduce the time and effort required for manual labelling tasks.
4. Real-Time Collaboration
For teams working on large projects, collaborating in real time is a game changer. This feature allows multiple users to work on the same dataset simultaneously, improving overall efficiency.
5. Integration Options
A good video labelling tool should seamlessly integrate with other platforms and tools. This ensures that your entire workflow, from data collection to model training, is coherent and streamlined.
The Role of KeyLabs.ai in Video Data Annotation
One platform that stands out in the realm of data annotation tools is KeyLabs.ai. Offering a comprehensive data annotation platform, KeyLabs.ai caters to various industry needs, including video labelling.
Why Choose KeyLabs.ai?
KeyLabs.ai has positioned itself as a leader in the data annotation space due to its unique offerings:
- Scalable Solutions: KeyLabs.ai provides scalable solutions that are adaptable to organizations of all sizes, from startups to large enterprises.
- High-Quality Annotation: Their team of expert annotators ensures that every piece of video data is accurately labelled, maintaining high standards of quality.
- Customizable Workflows: The platform allows for customization according to specific project requirements, giving users flexibility in how they approach their annotation tasks.
- Dedicated Support: KeyLabs.ai offers exceptional customer support, guiding users through the entire process of data annotation with professionalism and patience.
Use Cases for Video Labelling Tools
Video labelling tools provide immense value across numerous industries. Here are some specific use cases where these tools shine:
1. Autonomous Vehicles
The automotive sector utilizes video labelling extensively for training self-driving cars. Labels such as lane markings, pedestrians, and traffic signs are critical for the car’s ability to navigate safely.
2. Security and Surveillance
Security systems use video annotations to detect and categorize suspicious activities. Annotated video helps in the rapid identification of potential threats, improving safety measures.
3. Sports Analytics
In sports, video labelling allows coaches and analysts to break down game footage. Actions such as player movements, plays, and strategies can be meticulously labelled for post-game analysis and performance optimizations.
4. Medical Imaging
In healthcare, video labelling plays a crucial role in diagnosing conditions through video footage from procedures or examinations. Proper annotations help in training AI models to assist medical professionals.
Challenges in Video Data Annotation
Despite the advantages, there are challenges associated with the process of video data annotation. Here are a few hurdles organizations may face:
1. Volume of Data
The sheer volume of video data generated can be overwhelming. Managing and annotating large datasets requires significant resources and time.
2. Complexity of Annotations
Video content can be complex, with multiple objects, actions, and events occurring simultaneously. This complexity necessitates precise and often time-consuming labelling efforts.
3. Quality Assurance
Ensuring the quality and accuracy of annotations can be challenging, especially when relying on manual processes or less sophisticated tools.
Future Trends in Video Labelling
The future of video labelling tools is bright, reflecting the rapid advancements in AI and machine learning technologies. Here are expected trends:
1. Machine Learning Integration
More video labelling tools will leverage machine learning to enhance the speed and accuracy of annotation processes, allowing for semi-automated solutions.
2. Cloud-Based Solutions
The shift towards cloud-based annotation solutions will facilitate easier collaboration and access to resources from anywhere in the world.
3. Enhanced User Experience
As competition increases, user experience will continue to improve, with more intuitive interfaces and better support for various annotation styles.
Conclusion
In summary, video labelling tools are becoming an indispensable asset for any organization looking to harness the power of video data. Platforms like KeyLabs.ai offer robust solutions that can meet the demands of various industries. By understanding the importance of data annotation, selecting the right tool, and recognizing potential challenges, businesses can embark on their journey toward effective video data utilization. As the field evolves, those who adopt advanced video labelling technologies will undoubtedly have a competitive edge in their respective markets.